The Simplicity of Digital Intelligence
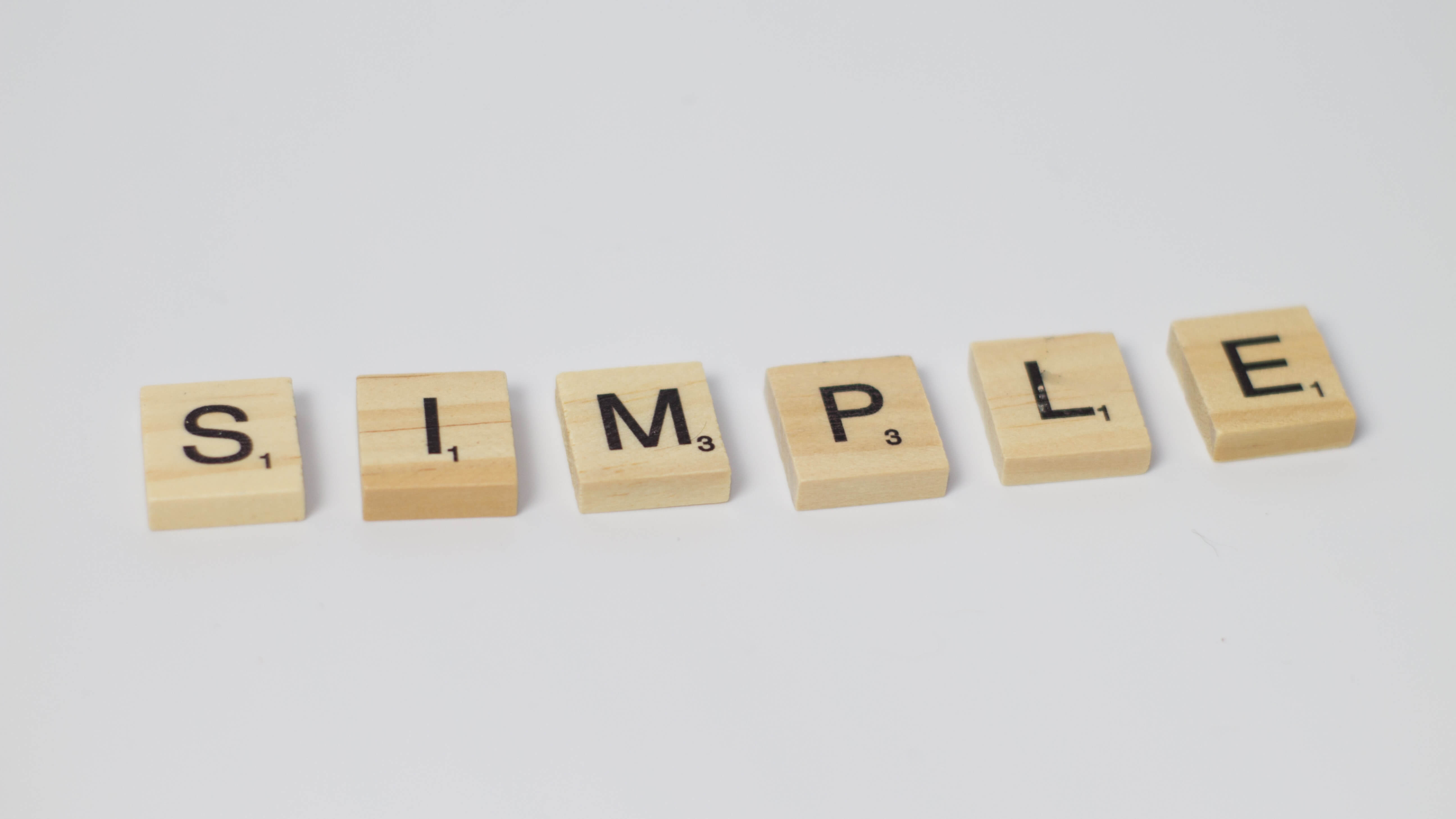
The year 1995 heralded the beginning of the new digital economy. It was the first time that the term ‘digital communication’ was used and set to upend markets and change everything. Many of us did not buy into that hype then. It wasn’t that we didn’t recognize that something changed. It was that we recognized that the complicated old ways would remain useful for looking at the changes taking place. The new economy - digital technology would cause a reduction in the cost of search and communication. This would lead to more searches, more communication, and more activities that go together with search and communications. That’s essentially what happened.
Today, we are seeing a similar trend in digital intelligence. But once again, we believe, we are there but not there yet, because we don’t know what that ‘there’ is. As in the past, simplicity - the ultimate sophistication comes to our aid. We know technological revolutions tend to involve some important activities becoming cheap, like the cost of communication or finding information. Digital Intelligence, in its essence, is an intersectional technology, a tool that will bring both the principles of digital transformation and business intelligence where the economic shift will centre around a drop in the cost of prediction.
In this blog, we, Celeix Digital, believe the first effect of digital intelligence will be to lower the cost of goods and services that rely on prediction. This matters because prediction is an input to a host of activities including transportation, agriculture, healthcare, energy manufacturing, and retail. When the cost of any input falls so precipitously, there are two well-established implications. First, we will start using prediction to perform tasks (business intelligence) and second, the value of other parameters that complement this prediction will rise (digital transformation).
Task-Oriented Business Intelligence
As Digital Intelligence lowers the cost of prediction, we will begin to use it as an input for purposes which we never previously did. As an example, consider semiconductors, an area of technological advance that caused a significant drop in the cost of different inputs - arithmetic. With semiconductors we could calculate cheaply, so activities for which arithmetic was a key input, such as data analysis and accounting, became much cheaper. However, we also started using the newly cheap arithmetic to solve problems that were not historically arithmetic problems. An example is the history of photography. We shifted from a film-oriented, chemistry-based approach to a digital-oriented, arithmetic-based approach. Other new applications for cheap arithmetic included communications, music, and drug discovery.
The same goes for Digital Intelligence and its prediction. As the cost of prediction falls, not only will activities that were historically prediction-oriented become cheaper — like inventory management and demand forecasting — but we will also use prediction to tackle other problems for which prediction was not historically an input. Consider navigation. Until recently, autonomous driving was limited to highly controlled environments such as warehouses and factories where programmers could anticipate the range of scenarios a vehicle may encounter, and could program if-then-else-type decision algorithms accordingly (for example - if an object approaches the vehicle, then slow down). It was inconceivable to put an autonomous vehicle on a city street because the number of possible scenarios in such an uncontrolled environment would require programming an almost infinite number of if-then-else statements. Inconceivable, that was, until recently. Once prediction became cheap, innovators reframed driving as a prediction problem, rather than programming endless if-then-else statements, we could instead simply use Digital Intelligence to predict — what would a human driver do?
We could outfit vehicles with a variety of sensors - cameras, lidar, radar, etc — and then collect millions of miles of human driving data. By linking the incoming environmental data from sensors on the outside of the car to the driving decisions (business intelligence) made by the human inside the car (steering, braking, accelerating - digital transformation), intersectional digital intelligence can be used to predict how humans would react to each second of incoming data about their environment. Thus, prediction is now a major component of the solution to a problem that was previously not considered a prediction problem.
Result-Oriented Digital Transformation
When the cost of a foundational input plummets, it often affects the value of other inputs. The value goes up for complements and down for substitutes. In the case of photography, the value of hardware and software components associated with digital cameras went up as the cost of arithmetic dropped because demand increased because we wanted more of them. These components were complements for arithmetic, the reason they were used together. In contrast, the value of film-related chemicals fell since we wanted less of them. All human activities can be described by five high-level components — data, prediction, judgement, action, and outcomes.
For example, a visit to the doctor in response to pain leads to x-rays, blood tests, and monitoring which is intelligence in the form of data. Diagnosis of the problem, such as, ‘if we administer treatment A, then we predict outcome X, but if we administer treatment B, then we predict outcome Y (prediction). The weighing options (given your age, lifestyle, and family status, I think you might be best with treatment a, let’s discuss how you feel about the risks and side effects (judgement) were the results of that administered intelligence in action leading to administering treatment A (action) and full recovery with minor side effects (outcome - the core principle of transformation).
As digital intelligence improves, the value of human prediction skills will decrease because business intelligence will provide a cheaper and better substitute for human intervention, just as machines did for arithmetic. However, this does not spell doom for human jobs. That’s because the value of human judgement skills will increase. This skill is a complement for prediction and therefore when the cost of prediction falls demand for judgement rises. We will want more human judgement. For example, when prediction is cheap, the diagnosis will be more frequent and convenient, and thus we will detect many more early-stage, treatable conditions. This will mean more decisions will be made about medical treatment, which means greater demand for the application of ethics, and more emotional support, which is provided by humans.
Digital Intelligence - A Judgement Call
The line between judgement and prediction isn’t clear cut - some judgement tasks will even be reframed as a series of predictions. Yet, overall the value of prediction-related human skills will fall, and the value of judgement-related skills will rise. Interpreting the rise of digital intelligence as a drop in the cost of prediction doesn’t offer an answer to every specific question of how the technology will play out. But it yields two key implications —
— an expanded role of prediction as an input to more goods and services and
— a change in the value of inputs, driven by the extent to which they are a complement of or substitutes for prediction.
At Celeix Digital we believe these changes are coming. The speed and extent to which Chief Digital Officers should invest in judgement-related capabilities will depend on how fast these changes arrive.
Transform Your Business with Digital Intelligence
Unlock the power of data and make strategic decisions with Celeix Digital's Digital Intelligence product