Harnessing the Power of Data - A Guide to Digital Intelligence in Business - Part Two
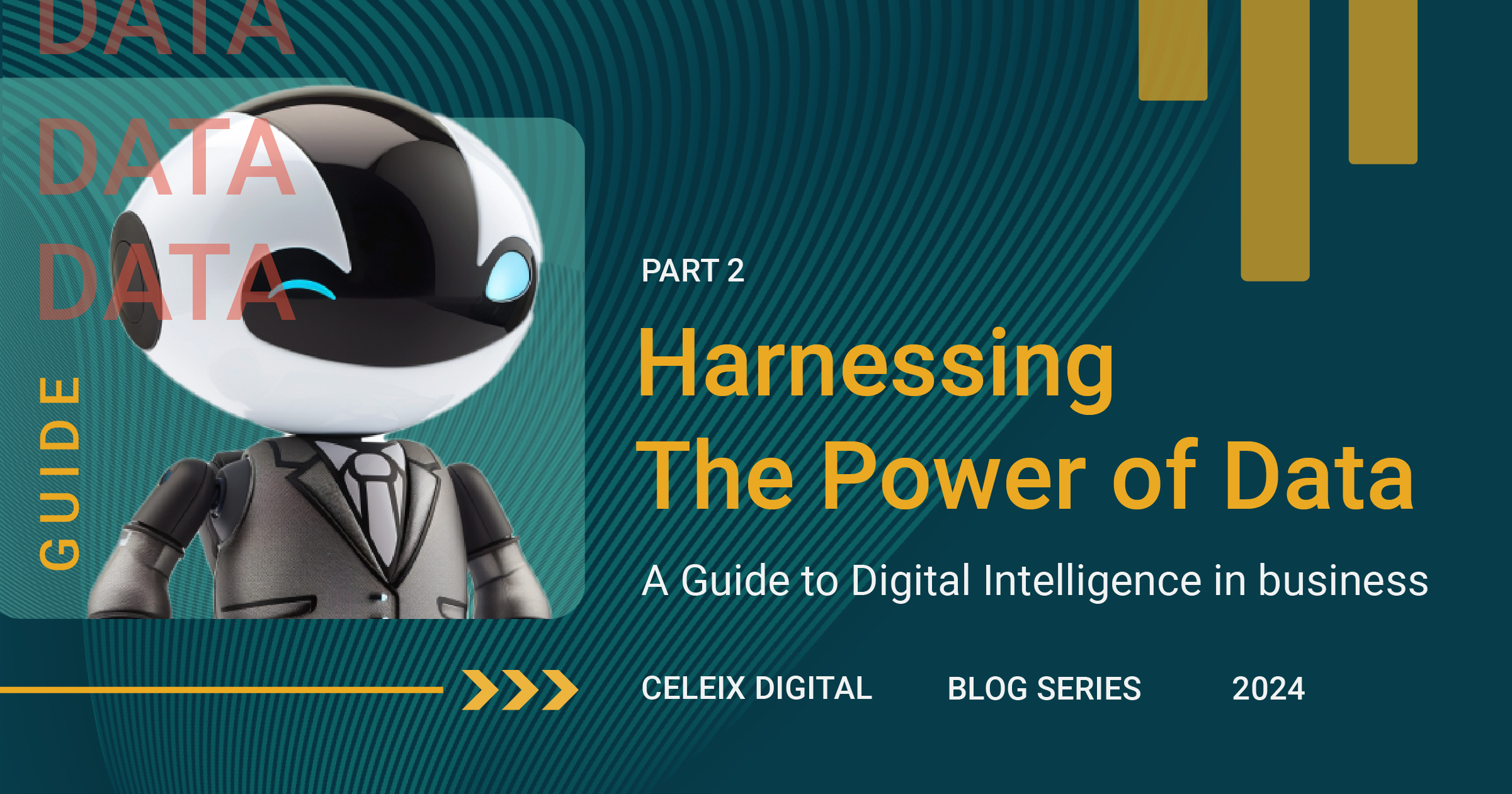
Key Methodologies - Data Mining, Statistical Analysis, and Data Visualisation
Data Mining
Data mining is a key methodology in business analytics that involves discovering patterns, relationships, and insights from large datasets. It encompasses various techniques such as association rule mining, classification, clustering, and anomaly detection. The goal of data mining is to extract actionable knowledge and uncover hidden patterns that can drive decision-making and business strategies. One widely used data mining technique is associated with rule mining, which identifies interesting relationships or associations among items in a dataset. A classic example is the market basket analysis, where associations between items frequently purchased together in a retail store are discovered. The Apriori algorithm, proposed by R. Agarwal and R. Srikant in 1994, is a popular algorithm for mining association rules. It efficiently identifies frequent item sets and generates rules based on user-defined support and confidence thresholds. Another essential data mining technique is classification, which involves assigning predefined labels or classes to new data instances based on their characteristics. Decision trees, neural networks and support vector machines are commonly employed algorithms for classifIcation tasks.
One seminal work in classification is the C4.5 algorithm, developed by J. Ross Quinlan in 1993. C4.5 constructs decision trees by recursively splitting the dataset based on attribute values, maximising information gain at each step. Additionally, clustering is a technique used to group similar data instances together based on their inherent characteristics. The k-means algorithm is a well-known clustering algorithm that partitions the dataset into ‘k’ clusters, aiming to minimise the within-cluster sum of squared distances. The algorithm iteratively updates the cluster centroids and assigns data points to the nearest centroid. J MacQueen proposed the k-means algorithm in 1967, which remains a fundamental technique in clustering.
Statistical Analysis
Statistical analysis plays a crucial role in business analytics, providing tools and techniques to analyse and interpret data, make inferences, and quantify uncertainty. Statistical analysis enables businesses to make data-driven decisions, validate hypotheses, and derive meaningful insights from data. One of the fundamental statistical techniques used in business analytics is regression analysis. Regression models examine the relationship between a dependent variable and one or more independent variables. Ordinary least squares (OLS) regression is a widely employed method for estimating the parameters of a linear regression model. It minimised the sum of squared differences between the observed and predicted values of the dependent variable. OLS regression was first introduced by C. F. Gauss in the early 19th century and remains a cornerstone of statistical analysis.
Another important statistical technique is hypothesis testing, which enables researchers to evaluate the significance of observed differences and relationships in data. The t-test is a commonly used hypothesis test for comparing means between two groups. It assesses whether the observed difference between group means is statistically different. The t-test was developed by W. S. Gosset (known as student) in 1908 and has since been widely applied in various fields. Furthermore, analysis of variance (ANOVA) is a statistical technique used to compare means across multiple groups, ANOVA assesses whether there are significant differences in means by analysing the variation between groups and within groups. R. A. Fisher developed anova in the early 20th century, revolutionising the field of experimental design and analysis.
Data Visualisation
Data visualisation is a critical aspect of business analytics that involves representing data graphically to facilitate understanding, exploration, and communication of insights. Effective data visualisation techniques help analysts and decision-makers gain valuable insights, identify patterns, and convey complex information intuitively. One widely uses data visualisation technique is the use of charts and graphs. Bar charts, line charts, scatter plots, and pie charts are commonly employed to depict relationships, trends, and distributions. Edward Tufte, a prominent expert in data visualisation, emphasised the importance of clear concise, and informative visual representations of data.
In recent years, interactive and dynamic visualisations have gained popularity. These techniques allow users to manipulate and explore data visually, uncovering insights interactively. Interactive dashboards, network visualisations, and geographic maps with filtering and drill-down capabilities enable users to dive deeper into data and discover hidden patterns. D3.js (Data-Driven Documents), a JavaScript library developed by M. Bostock et al, has played a significant role in advancing interactive data visualisation on the web. In summary, data mining, statistical analysis, and data visualisation are key methodologies in business analytics that provide valuable tools nad techniques for extracting insights, making informed decisions, and communicating findings effectively. These methodologies form the foundation of data-driven decision-making and have contributed to numerous advancements in various industries.
At Celeix Digital, we believe the roadmap extends far beyond its origins for data and is nowhere near its destination yet.
Agrawal, R., & Srikant, R. (1994). Fast Algorithms for Mining Association Rules in Large Databases Proceedings of the 20th International Conference on Very Large Data Bases, Aliahmadi, A., Nozari, H., & Ghahremani-Nahr, J. (2022a). AIoT-based Sustainable Smart Supply Chain Framework. International Journal of Innovation in Management, Economics and Social Sciences, 2(2), 28-38. https://doi.org/10.52547/ijimes.2.2.28
Aliahmadi, A., Nozari, H., & Ghahremani-Nahr, J. (2022b). Big Data IoT-based Agile-Lean Logistic in Pharmaceutical Industries. International Journal of Innovation in Management, Economics and Social Sciences, 2(3), 70-81. https://doi.org/10.52547/ijimes.2.3.70
Alnoukari, M. (2022). From Business Intelligence to Big Data: The Power of Analytics. In I. R. Management Association (Ed.), Research Anthology on Big Data Analytics, Architectures, and Applications (pp. 823-841). IGI Global. https://doi.org/10.4018/978-1-6684-3662-2.ch038
Barocas, S., & Selbst, A. D. (2016). Big Data's Disparate Impact. California Law Review, 104(3), 671-732. http://www.jstor.org/stable/24758720
Barredo Arrieta, A., Díaz-Rodríguez, N., Del Ser, J., Bennetot, A., Tabik, S., Barbado, A., Garcia, S., Gil-Lopez, S., Molina, D., Benjamins, R., Chatila, R., & Herrera, F. (2020). Explainable Artificial Intelligence (XAI): Concepts, taxonomies, opportunities and challenges toward responsible AI. Information Fusion, 58, 82-115. https://doi.org/https://doi.org/10.1016/j.inffus.2019.12.012
Bishop, C. M. (2006). Pattern Recognition and Machine Learning. Springer. https://books.google.com/books?id=qWPwnQEACAAJ
Bojarski, M., Testa, D., Dworakowski, D., Firner, B., Flepp, B., Goyal, P., Jackel, L., Monfort, M., Muller, U., Zhang, J., Zhang, X., Zhao, J., & Zieba, K. (2016). End to End Learning for Self-Driving Cars. https://doi.org/https://doi.org/10.48550/arXiv.1604.07316
Bostock, M., Ogievetsky, V., & Heer, J. (2011). D3 Data-Driven Documents. IEEE Transactions on Visualization and Computer Graphics, 17(12), 2301-2309. https://doi.org/10.1109/TVCG.2011.185
Brose, M. S., Flood, M. D., Krishna, D., & Nichols, B. (2014). Handbook of Financial Data and Risk Information I: Principles and Context (M. S. Brose, M. D. Flood, D. Krishna, & B. Nichols, Eds. Vol. 1). Cambridge University Press. https://doi.org/DOI: 10.1017/CBO9780511997723
Bruun, E. P. G., & Duka, A. (2018). Artificial Intelligence, Jobs and the Future of Work: Racing with the Machines. Basic Income Studies, 13(2). https://doi.org/doi:10.1515/bis-2018-0018
Brynjolfsson, E., & McAfee, A. (2017). The business of artificial intelligence. Harvard Business Review,, 95(1), 56-66. https://hbr.org/2017/07/the-business-of-artificial-intelligence
Bughin, J., Chui, M., & Manyika, J. (2018). Notes from the AI frontier: Insights from hundreds of use cases. M. G. Institute. Caliskan, A., Bryson, J. J., & Narayanan, A. (2017). Semantics derived automatically from language corpora contain human-like biases. Science, 356(6334), 183-186. https://doi.org/10.1126/science.aal4230
Campbell, C., Sands, S., Ferraro, C., Tsao, H.-Y., & Mavrommatis, A. (2020). From data to action: How marketers can leverage AI. Business Horizons, 63(2), 227-243. https://doi.org/https://doi.org/10.1016/j.bushor.2019.12.002
Cavoukian, A. (2018). Privacy by design: The 7 foundational principles. https://iapp.org/media/pdf/knowledge_center/Privacy-by-Design-The-7-Fundamental-Principles.pdf
Chen, H., Chiang, R. H. L., & Storey, V. C. (2012). Business Intelligence and Analytics: From Big Data to Big Impact. MIS Quarterly, 36(4), 1165-1188. https://doi.org/10.2307/41703503
Chen, I. Y., Johansson, F. D., & Sontag, D. A. (2018). Why Is My Classifier Discriminatory? Neural Information Processing Systems, Chen, P.-T., Lin, C.-L., & Wu, W.-N. (2020). Big data management in healthcare: Adoption challenges and implications. International Journal of Information Management, 53, 102078. https://doi.org/https://doi.org/10.1016/j.ijinfomgt.2020.102078
Chen, X., Kundu, K., Zhu, Y., Berneshawi, A., Ma, H., Fidler, S., & Urtasun, R. (2015). 3D object proposals for accurate object class detection Proceedings of the 28th International Conference on Neural Information Processing Systems - Volume 1, Montreal, Canada. Cohen, M. C. (2018). Big Data and Service Operations. Production and Operations Management, 27(9), 1709-1723. https://doi.org/https://doi.org/10.1111/poms.12832
Corbett-Davies, S., & Goel, S. (2018). The Measure and Mismeasure of Fairness: A Critical Review of Fair Machine Learning. ArXiv, abs/1808.00023. Davenport, T. H., & Harris, J. G. (2007). Competing on analytics. Harvard Business Review, 85(1), 98-107. https://hbr.org/2006/01/competing-on-analytics
Davenport, T. H., & Patil, D. J. (2012). Data Scientist: The Sexiest Job of the 21st Century Harvard Business Review, 90(10), 70-76. https://hbr.org/2012/10/data-scientist-the-sexiest-job-of-the-21st-century Delen, D., & Ram, S. (2018). Research challenges and opportunities in business analytics. Journal of Business Analytics, 1(1), 2-12. https://doi.org/10.1080/2573234X.2018.1507324
Demajo, L., Vella, V., & Dingli, A. (2020). Explainable AI for Interpretable Credit Scoring. https://doi.org/10.5121/csit.2020.101516
Devlin, J., Chang, M.-W., Lee, K., & Toutanova, K. (2019). BERT: Pre-training of Deep Bidirectional Transformers for Language Understanding. North American Chapter of the Association for Computational Linguistics, Dubey, R., Gunasekaran, A., Childe, S. J., Roubaud, D., Fosso Wamba, S., Giannakis, M., & Foropon, C. (2019). Big data analytics and organizational culture as complements to swift trust and collaborative performance in the humanitarian supply chain. International Journal of Production Economics, 210, 120-136. https://doi.org/https://doi.org/10.1016/j.ijpe.2019.01.023
European-Union. (2016). Regulation (EU) 2016/679 of the European Parliament and of the Council of 27 April 2016 on the protection of natural persons with regard to the processing of personal data and on the free movement of such data, and repealing Directive 95/46/EC (General Data Protection Regulation). . L. Official Journal of the European Union. http://data.europa.eu/eli/reg/2016/679/oj
Fan, W., & Geerts, F. (2012). Foundations of Data Quality Management. https://doi.org/10.1007/978-3-031-01892-3
Fisher, R. A. (1925). Statistical Methods for Research Workers. Oliver and Boyd. https://books.google.com/books?id=3C7QAAAAMAAJ
Floridi, L., Cowls, J., Beltrametti, M., Chatila, R., Chazerand, P., Dignum, V., Luetge, C., Madelin, R., Pagallo, U., Rossi, F., Schafer, B., Valcke, P., & Vayena, E. (2021). An Ethical Framework for a Good AI Society: Opportunities, Risks, Principles, and Recommendations. In L. Floridi (Ed.), Ethics, Governance, and Policies in Artificial Intelligence (pp. 19-39). Springer International Publishing. https://doi.org/10.1007/978-3-030-81907-1_3
Fosso Wamba, S., Akter, S., Edwards, A., Chopin, G., & Gnanzou, D. (2015). How ‘big data’ can make big impact: Findings from a systematic review and a longitudinal case study. International Journal of Production Economics, 165, 234-246. https://doi.org/https://doi.org/10.1016/j.ijpe.2014.12.031
Frey, C. B., & Osborne, M. A. (2017). The future of employment: How susceptible are jobs to computerisation? Technological Forecasting and Social Change, 114, 254-280. https://doi.org/https://doi.org/10.1016/j.techfore.2016.08.019
Gandomi, A., & Haider, M. (2015). Beyond the hype: Big data concepts, methods, and analytics. International Journal of Information Management, 35(2), 137-144. https://doi.org/https://doi.org/10.1016/j.ijinfomgt.2014.10.007
Gauss, C. F., & Gottingensis, S. R. S. (1821). Theoria combinationis observationum: erroribus minimis obnoxiae. https://books.google.com/books?id=4BrCswEACAAJ
Ghahremani nahr, J., Nozari, H., & Sadeghi, M. E. (2021a). Artificial intelligence and Machine Learning for Real- world problems (A survey). International Journal of Innovation in Engineering, 1(3), 38-47. https://doi.org/10.59615/ijie.1.3.38
Ghahremani Nahr, J., Nozari, H., & Sadeghi, M. E. (2021b). Green supply chain based on artificial intelligence of things (AIoT). International Journal of Innovation in Management, Economics and Social Sciences, 1(2), 56-63. https://doi.org/10.52547/ijimes.1.2.56
Goodman, B., & Flaxman, S. (2017). European Union Regulations on Algorithmic Decision Making and a “Right to Explanation”. AI Magazine, 38(3), 50-57. https://doi.org/https://doi.org/10.1609/aimag.v38i3.2741
hajiaghajani, A. (2023). Concepts and applications of data mining and analysis of social networks. Journal of Data Analytics, 2(1), 1-8. https://doi.org/10.59615/jda.2.1.1
Hastie, T., Tibshirani, R., & Friedman, J. (2009). The Elements of Statistical Learning: Data Mining, Inference, and Prediction, Second Edition. Springer New York. https://books.google.com/books?id=tVIjmNS3Ob8C
He, K., Zhang, X., Ren, S., & Sun, J. (2016, 27-30 June 2016). Deep Residual Learning for Image Recognition. 2016 IEEE Conference on Computer Vision and Pattern Recognition (CVPR), Hochreiter, S., & Schmidhuber, J. (1997). Long Short-Term Memory. Neural Computation, 9(8), 1735-1780. https://doi.org/10.1162/neco.1997.9.8.1735
James, G., Witten, D., Hastie, T., & Tibshirani, R. (2013). An Introduction to Statistical Learning: with Applications in R. Springer New York. https://books.google.com/books?id=qcI_AAAAQBAJ
Jędrzejka, D. (2019). Robotic process automation and its impact on accounting. Zeszyty Teoretyczne Rachunkowości, 2019, 137-166. https://doi.org/10.5604/01.3001.0013.6061
John, A., & Latha, T. (2023). Stock market prediction based on deep hybrid RNN model and sentiment analysis. Automatika, 64(4), 981-995. https://doi.org/10.1080/00051144.2023.2217602
Kaplan, R. S., & Norton, D. P. (1992). The balanced scorecard: Measures that drive performance. Harvard Business Review, 70(1), 71-79. https://hbr.org/1992/01/the-balanced-scorecard-measures-that-drive-performance-2
Kavanagh, M. J., Thite, M., & Johnson, R. D. (2011). Human Resource Information Systems: Basics, Applications, and Future Directions: Basics, Applications, and Future Directions. SAGE Publications. https://books.google.com/books?id=yDe-rMyZSbkC
Kian, R. (2021). Provide a model for an e-commerce system with the impact of artificial intelligence. International Journal of Innovation in Management, Economics and Social Sciences, 1(3), 88-94. https://doi.org/10.52547/ijimes.1.3.88
Kim, Y. (2014). Convolutional Neural Networks for Sentence Classification. Proceedings of the 2014 Conference on Empirical Methods in Natural Language Processing, 1746–1751. https://doi.org/10.3115/v1/D14-1181
Kober, J., Bagnell, J. A., & Peters, J. (2013). Reinforcement learning in robotics: A survey. The International Journal of Robotics Research, 32(11), 1238-1274. https://doi.org/10.1177/0278364913495721
Krizhevsky, A., Sutskever, I., & Hinton, G. E. (2012). ImageNet classification with deep convolutional neural networks. In F. Pereira, C. J. Burges, L. Bottou, & K. Q. Weinberger (Eds.), Advances in Neural Information Processing Systems (Vol. 25). Larson, D., & Chang, V. (2016). A review and future direction of agile, business intelligence, analytics and data science. International Journal of Information Management, 36(5), 700-710. https://doi.org/https://doi.org/10.1016/j.ijinfomgt.2016.04.013
Lavalle, S., Lesser, E., Shockley, R., Hopkins, M., & Kruschwitz, N. (2011). Big Data, Analytics and the Path From Insights to Value. MIT Sloan Management Review, 52, 21-32. https://sloanreview.mit.edu/article/big-data-analytics- and-the-path-from-insights-to-value/
LeCun, Y., Bengio, Y., & Hinton, G. (2015). Deep learning. Nature, 521(7553), 436-444. https://doi.org/10.1038/nature14539
Lee, I., & Mangalaraj, G. (2022). Big Data Analytics in Supply Chain Management: A Systematic Literature Review and Research Directions. Big Data and Cognitive Computing, 6(1), 17. https://www.mdpi.com/2504-2289/6/1/17
Levine, S., Finn, C., Darrell, T., & Abbeel, P. (2015). End-to-End Training of Deep Visuomotor Policies. Journal of Machine Learning Research, 17(39), 1-40. http://jmlr.org/papers/v17/15-522.html
Li, L., Chi, T., Hao, T., & Yu, T. (2018). Customer demand analysis of the electronic commerce supply chain using Big Data. Annals of Operations Research, 268(1), 113-128. https://doi.org/10.1007/s10479-016-2342-x
Liebowitz, J. (2013). Business Analytics: An Introduction (1st ed.). CRC Press. https://doi.org/https://doi.org/10.1201/b16246
Lipton, Z. C. (2018). The Mythos of Model Interpretability: In machine learning, the concept of interpretability is both important and slippery. Queue, 16(3), 31–57. https://doi.org/10.1145/3236386.3241340
MacQueen, J. (1967). Some methods for classification and analysis of multivariate observations. In L. M. L. Cam & J. Neyman (Eds.), Proceedings of the Fifth Berkeley Symposium on Mathematical Statistics and Probability. University of California Press. https://books.google.com/books?id=IC4Ku_7dBFUC
Maleki, R., & Sabet, E. (2022). Business Intelligence Analysis in Small and Medium Enterprises. International journal of Innovation in Marketing Elements, 2(1), 1-11. https://doi.org/10.59615/ijime.2.1.1
McCarthy, J., Minsky, M., Shannon, C. E., Rochester, N., & Dartmouth, C. (1955). A proposal for the Dartmouth Summer Research Project on Artificial Intelligence. Merchán, D., Arora, J., Pachon, J., Konduri, K., Winkenbach, M., Parks, S., & Noszek, J. (2022). 2021 Amazon Last Mile Routing Research Challenge: Data Set. Transportation Science. https://doi.org/10.1287/trsc.2022.1173
Miotto, R., Li, L., Kidd, B. A., & Dudley, J. T. (2016). Deep Patient: An Unsupervised Representation to Predict the Future of Patients from the Electronic Health Records. Scientific Reports, 6(1), 26094. https://doi.org/10.1038/srep26094
Mirowski, P., Pascanu, R., Viola, F., Soyer, H., Ballard, A., Banino, A., Denil, M., Goroshin, R., Sifre, L., Kavukcuoglu, K., Kumaran, D., & Hadsell, R. (2016). Learning to Navigate in Complex Environments. https://doi.org/https://doi.org/10.48550/arXiv.1611.03673
Mitchell, T. M. (1997). Machine Learning. McGraw-Hill Education. https://books.google.com/books?id=xOGAngEACAAJ
Mohseni Kiasari, M., & Fartash, K. (2023). Prioritizing policy tools to support development of IoT technologies in Iran. International Journal of Innovation in Engineering, 3(2), 53-67. https://ijie.ir/index.php/ijie/article/view/121
Mortaji, S. T. H., Noori, S., & Bagherpour, M. (2021a). Adaptive Project Monitoring and Control with Variable Reviewing Intervals. International Journal of Innovation in Engineering, 1(4), 34-46. https://doi.org/10.59615/ijie.1.4.34
Mortaji, S. T. H., Noori, S., & Bagherpour, M. (2021b). Directed earned value management based on ordered fuzzy numbers. Journal of Intelligent & Fuzzy Systems, 40, 10183-10196. https://doi.org/10.3233/JIFS-201248
Mortaji, S. T. H., Noori, S., Noorossana, R., & Bagherpour, M. (2018). An ex ante control chart for project monitoring using earned duration management observations. Journal of Industrial Engineering International, 14(4), 793-806. https://doi.org/10.1007/s40092-017-0251-5
Mortaji, S. T. H., Noorossana, R., & Bagherpour, M. (2015). Project Completion Time and Cost Prediction Using Change Point Analysis. Journal of Management in Engineering, 31(5), 04014086. https://doi.org/doi:10.1061/(ASCE)ME.1943-5479.0000329
Moslemi, S. (2021). Examining the Dimensions of Big Data Privacy (Block Chain Solution for Privacy Protection). International Journal of Innovation in Management, Economics and Social Sciences, 1(4), 10-16. https://doi.org/10.52547/ijimes.1.4.10
Mousakhani, M., Saghafi, F., Hasanzadeh, M., & Sadeghi, M. E. (2020). Proposing Dynamic Model of Functional Interactions of IoT Technological Innovation System by Using System Dynamics and Fuzzy DEMATEL [Research]. Journal of Operational Research and Its Applications, 17(4), 1-21. http://jamlu.liau.ac.ir/article-1-1869-en.html
nazari, E., Edalati Khodabandeh, M., Dadashi, A., Aldaghi, T., Rasoulian, M., & Tabesh, H. (2022). Studying Students' Knowledge of the Benefits, Challenges, and Applications of Big Data Analytics in Healthcare. International Journal of Innovation in Engineering, 2(1), 40-57. https://doi.org/10.59615/ijie.2.1.40
Nilsson, N. J. (1998). Artificial Intelligence: A New Synthesis. Morgan Kaufmann Publishers. https://books.google.com/books?id=GYOFSd6fETgC
Nozari, H., Ghahremani-Nahr, J., Fallah, M., & Szmelter-jarosz, A. (2022). Assessment of Cyber Risks in an IoT- based Supply Chain using a Fuzzy Decision-Making Method. International Journal of Innovation in Management, Economics and Social Sciences, 2(1), 52-64. https://doi.org/10.52547/ijimes.2.1.52
Nozari, H., Sadeghi, M. E., & Najafi, S. E. (2022). Quantitative Analysis of Implementation Challenges of IoT-Based Digital Supply Chain (Supply Chain 0/4). arXiv preprint arXiv:2206.12277.
Obaid, H. S. (2022). Review the challenges of using big data in the supply chain. Journal of Data Analytics, 1(1), 16-24. https://doi.org/10.59615/jda.1.1.16
Popat, R. R., & Chaudhary, J. (2018, 11-12 May 2018). A Survey on Credit Card Fraud Detection Using Machine Learning. 2018 2nd International Conference on Trends in Electronics and Informatics (ICOEI), Power, D. (2002). Decision Support Systems: Concepts and Resources for Managers. Bloomsbury Academic. https://books.google.com/books?id=9NA6QMcte3cC
Prat, N. (2019). Augmented Analytics. Business & Information Systems Engineering, 61(3), 375-380. https://doi.org/10.1007/s12599-019-00589-0 Pumsirirat, A., & Yan, L. (2018). Credit Card Fraud Detection using Deep Learning based on Auto-Encoder and Restricted Boltzmann Machine. International Journal of Advanced Computer Science and Applications, 9(1). https://doi.org/http://dx.doi.org/10.14569/IJACSA.2018.090103
Quinlan, J. R. (1993). C4.5: Programs for Machine Learning. Elsevier Science. https://doi.org/https://doi.org/10.1016/C2009-0-27846-9
Rahmaty, M. (2023). Machine learning with big data to solve real-world problems. Journal of Data Analytics, 2(1), 9- 16. https://doi.org/10.59615/jda.2.1.9
Rajaraman, A., & Ullman, J. D. (2011). Mining of Massive Datasets. Cambridge University Press. https://doi.org/DOI: 10.1017/CBO9781139058452
Rajpurkar, P., Irvin, J., Ball, R. L., Zhu, K., Yang, B., Mehta, H., Duan, T., Ding, D., Bagul, A., Langlotz, C. P., Patel, B. N., Yeom, K. W., Shpanskaya, K., Blankenberg, F. G., Seekins, J., Amrhein, T. J., Mong, D. A., Halabi, S. S., Zucker, E. J., . . . Lungren, M. P. (2018). Deep learning for chest radiograph diagnosis: A retrospective comparison of the CheXNeXt algorithm to practicing radiologists. PLOS Medicine, 15(11), e1002686. https://doi.org/10.1371/journal.pmed.1002686
Ransbotham, S., Kiron, D., Gerbert, P., & Reeves, M. (2017). Reshaping business with artificial intelligence: Closing the gap between ambition and action. MIT Sloan Management Review, 59(1). Ren, S., He, K., Girshick, R., & Sun, J. (2015). Faster R-CNN: towards real-time object detection with region proposal networks Proceedings of the 28th International Conference on Neural Information Processing Systems - Volume 1, Montreal, Canada. Roman, R., Lopez, J., & Mambo, M. (2018). Mobile edge computing, Fog et al.: A survey and analysis of security threats and challenges. Future Generation Computer Systems, 78, 680-698. https://doi.org/https://doi.org/10.1016/j.future.2016.11.009
Russell, S., & Norvig, P. (2016). Artificial Intelligence: A Modern Approach. CreateSpace Independent Publishing Platform. https://books.google.com/books?id=PQI7vgAACAAJ
Sabet, E. (2021). Reviewing the Challenges of Big Data Use in Smart Industries. International Journal of Innovation in Management, Economics and Social Sciences, 1(4), 66-72. https://doi.org/10.52547/ijimes.1.4.66
Sadeghi, M. E. (2022). Big data based on IoT in the agriculture industry: developments, opportunities, and challenges ahead. Journal of Data Analytics, 1(1), 25-32. https://doi.org/10.59615/jda.1.1.25
Sadeghi, M. E., & Jafari, H. (2021). Investigating the dimensions, components and key indicators of supply chain management based on digital technologies. International Journal of Innovation in Management, Economics and Social Sciences, 1(3), 82-87. https://doi.org/10.52547/ijimes.1.3.82
Samadi-Parviznejad, P. (2022). The role of big data in digital transformation. Journal of Data Analytics, 1(1), 42-47. https://doi.org/10.59615/jda.1.1.42
Schroff, F., Kalenichenko, D., & Philbin, J. (2015, 7-12 June 2015). FaceNet: A unified embedding for face recognition and clustering. 2015 IEEE Conference on Computer Vision and Pattern Recognition (CVPR), Shahvaroughi Farahani, M., & Esfahani, A. (2022). Opportunities and Challenges of Applying Artificial Intelligence in the Financial Sectors and Startups during the Coronavirus Outbreak. International Journal of Innovation in Management, Economics and Social Sciences, 2(4), 33-55. https://doi.org/10.52547/ijimes.2.4.33
Shahvaroughi Farahani, M., Esfahani, A., Nejad Falatouri Moghaddam, M., & Ramezani, A. (2022). The Impact of Fintech and Artificial Intelligence on COVID 19 and Sustainable Development Goals. International Journal of Innovation in Management, Economics and Social Sciences, 2(3), 14-31. https://doi.org/10.52547/ijimes.2.3.14
Sharda, R., Turban, E., Delen, D., Aronson, J. E., Liang, T. P., & King, D. (2014). Business Intelligence and Analytics: Systems for Decision Support. Pearson. https://books.google.com/books?id=FLYDnwEACAAJ
Simonyan, K., & Zisserman, A. (2014). Very deep convolutional networks for large-scale image recognition. arXiv preprint arXiv:1409.1556. Souza, R. M., Nascimento, E. G. S., Miranda, U. A., Silva, W. J. D., & Lepikson, H. A. (2021). Deep learning for diagnosis and classification of faults in industrial rotating machinery. Computers & Industrial Engineering, 153, 107060. https://doi.org/https://doi.org/10.1016/j.cie.2020.107060
Student. (1908). The Probable Error of a Mean. Biometrika, 6(1), 1-25. https://doi.org/10.2307/2331554
Sutskever, I., Vinyals, O., & Le, Q. V. (2014). Sequence to Sequence Learning with Neural Networks http://papers.nips.cc/paper/5346-sequence-to-sequence-learning-with-neural-networks
Sutton, R. S., & Barto, A. G. (2018). Reinforcement Learning, second edition: An Introduction. MIT Press. https://books.google.com/books?id=sWV0DwAAQBAJ
Tufte, E. R. (1983). The Visual Display of Quantitative Information. Graphics Press. https://books.google.com/books?id=SqVpAAAAMAAJ
Vaswani, A., Shazeer, N. M., Parmar, N., Uszkoreit, J., Jones, L., Gomez, A. N., Kaiser, L., & Polosukhin, I. (2017). Attention is All you Need. Neural Information Processing Systems, Vergara, J. R., & Estévez, P. A. (2014). A review of feature selection methods based on mutual information. Neural Computing and Applications, 24(1), 175-186. https://doi.org/10.1007/s00521-013-1368-0
Verhoef, P. C., Kannan, P. K., & Inman, J. J. (2015). From Multi-Channel Retailing to Omni-Channel Retailing: Introduction to the Special Issue on Multi-Channel Retailing. Journal of Retailing, 91(2), 174-181. https://doi.org/https://doi.org/10.1016/j.jretai.2015.02.005
World-Economic-Forum. (2020). The future of jobs report 2020. W. E. Forum. http://www3.weforum.org/docs/WEF_Future_of_Jobs_2020.pdf
Zarsky, T. Z. (2019). Privacy and Manipulation in the Digital Age. Theoretical Inquiries in Law, 20(1), 157-188. https://doi.org/doi:10.1515/til-2019-0006
Transform Your Business with Digital Intelligence
Unlock the power of data and make strategic decisions with Celeix Digital's Digital Intelligence product